Lars Schaaf
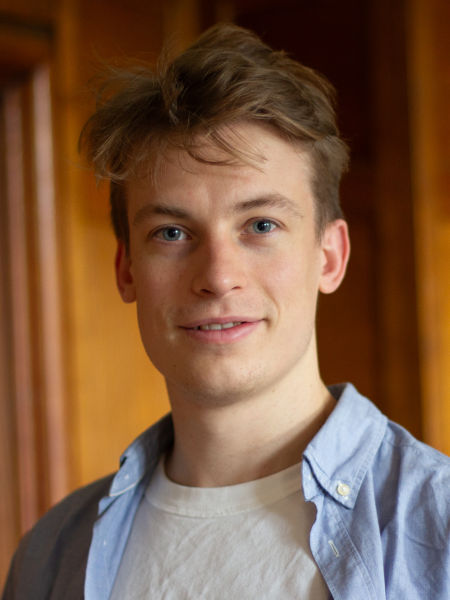
Mr Lars Schaaf
Fellow of Wolfson College
Postdoc in Dr Schran's group
Office: C3.068
Personal web site
Google Scholar
TCM Group, Cavendish Laboratory
Ray Dolby Centre,
JJ Thomson Avenue,
Cambridge, CB3 0US UK.
Research
Quantum mechanical calculations which directly solve the Schrodinger (or Dirac) equation have proved to be very powerful in explaining and predicting the properties of materials in many fields, including engineering, electro-chemistry, biology and medicine. They have also been effective at discovering novel candidate materials for a wide range of applications. However, they can be extremely computationally expensive, particularly so if more than a couple of hundred atoms need to be simulated.
Machine learning can enormously accelerate the calculations, enabling many more candidates to be screened, and calculations on far larger systems. One area of interest is the development of machine learning techniques which better model non-local interactions. Potential areas of application include drug design, rechargeable battery technologies, and catalysis.
In Plain English
From drug design to engineering, experiments are slow and expensive. When looking for a new drug or material, it can be much quicker and cheaper to perform initial "experiments" by computer simulation, and then perform real experiments only on those candidates whose calculated properties are promising.
But even computer simulation can be slow and expensive if one attempts an accurate solution of the equations of quantum mechanics. I develop and apply novel machine learning techniques to approximate full quantum mechanical calculations, but to do so thousands of times faster.